2012-02-21 by Stefan Urbanek
What is inside the Cubes Python OLAP Framework? Here is a brief overview of the core modules, their purpose and functionality.
The lightweight framework Cubes is composed of four public modules:

- model - Description of data (metadata): dimensions, hierarchies, attributes, labels, localizations.
- browser - Aggregation browsing, slicing-and-dicing, drill-down.
- backends - Actual aggregation implementation and utility functions.
- server - WSGI HTTP server for Cubes
Model
Logical model describes the data from user’s or analyst’s perspective: data how they are being measured, aggregated and reported. Model is independent of physical implementation of data. This physical independence makes it easier to focus on data instead on ways of how to get the data in understandable form.
Cubes model is described by:

- model object (doc)
- list of cubes
- dimensions of cubes (they are shared with all cubes within model) (doc) (doc)
- hierarchies (doc) and hierarchy levels (doc) of dimensions (such as category-subcategory, country-region-city)
- optional mappings from logical model to the physical model (doc)
- optional join specifications for star schemas, used by the SQL denormalizing backend (doc)
There is a utility function provided for loading the model from a JSON file: load_model
.
The model module object are capable of being localized (see Model Localization for more information). The cubes provides localization at the metadata level (the model) and functionality to have localization at the data level.
See also: Model Documentation
Browser
Core of the Cubes analytics functionality is the aggregation browser. The browser
module contains utility classes and functions for the browser to work.

The module components are:
- Cell – specification of the portion of the cube to be explored, sliced or drilled down. Each cell is specified by a set of cuts. A cell without any cuts represents whole cube.
- Cut – definition where the cell is going to be sliced through single dimension. There are three types of cuts: point, range and set.
The types of cuts:
- Point Cut – Defines one single point on a dimension where the cube is going to be sliced. The point might be at any level of hierarchy. The point is specified by "path". Examples of point cut:
[2010]
for year level of Date dimension, [2010,1,7]
for full date point.
- Range Cut – Defines two points (dimension paths) on a sortable dimension between whose the cell is going to be sliced from cube.
- Set Cut – Defines list of multiple points (dimension paths) which are going to be included in the sliced cell.
Example of point cut effect:

The module provides couple utility functions:
path_from_string
- construct a dimension path (point) from a string
string_from_path
- get a string representation of a dimension path (point)
string_from_cuts
and cuts_from_string
are for conversion between string and list of cuts. (Currently only list of point cuts are supported in the string representation)
The aggregation browser can:
- aggregate a cell (
aggregate(cell)
)
- drill-down through multiple dimensions and aggregate (
aggregate(cell, drilldown="date")
)
- get all detailed facts within the cell (
facts(cell)
)
- get single fact (
fact(id)
)
There is convenience function report(cell, report)
that can be implemented by backend in more efficient way to get multiple aggregation queries in single call.
More about aggregated browsing can be found in the Cubes documentation.
Backends
Actual aggregation is provided by the backends. The backend should implement aggregation browser interface.

Cubes comes with built-in ROLAP backend which uses SQL database through SQLAlchemy. The backend has two major components:
- aggregation browser that works on single denormalized view or a table
- SQL denormalizer helper class that converts star schema into a denormalized view or table (kind of materialisation).
There was an attempt to write a Mongo DB backend, but it does not work any more, it is included in the sources only as reminder, that there should be a mongo backend sometime in the future.
Anyone can write a backend. If you are interested, drop me a line.
Server
Cubes comes with Slicer - a WSGI HTTP OLAP server with API for most of the cubes framework functionality. The server is based on the Werkzeug framework.

Intended use of the slicer is basically as follows:
- application prepares the cell to be aggregated, drilled, listed... The cell might be whole cube.
- HTTP request is sent to the server
- the server uses appropriate aggregation browser backend (note that currently there is only one: SQL denormalized) to compute the request
- Slicer returns a JSON reply to the application
For more information, please refer to the Cubes Slicer server documentation.
One more thing...
There are plenty things to get improved, of course. Current focus is not on performance, but on achieving simple usability.
The Cubes sources can be found on Github: https://github.com/stiivi/cubes . There is also a IRC channel #databrewery on irc.freenode.net (I try to be there during late evening CET). Issues can be reported on the github project page.
If you have any questions, suggestions, recommendations, just let me know.
HackerNews Thread
2011-11-28 by Stefan Urbanek
In this Cubes OLAP how-to we are going to learn:
- how to create a hierarchical dimension
- how to do drill-down through a hierarchy
- detailed level description
In the previous
tutorial we learned how
to use model descriptions in a JSON file and how to do physical to logical mappings.
Data used are similar as in the second tutorial, manually modified IBRD Balance
Sheet taken
from The World
Bank.
Difference between second tutorial and this one is added two columns: category code and sub-category code.
They are simple letter codes for the categories and subcategories.
Hierarchy
Some dimensions can have multiple levels forming a hierarchy. For example dates have year, month, day;
geography has country, region, city; product might have category, subcategory and the product.
Note: Cubes supports multiple hierarchies, for example for date you might have year-month-day or
year-quarter-month-day. Most dimensions will have one hierarchy, thought.
In our example we have the item
dimension with three levels of hierarchy: category, subcategory and
line item:

The levels are defined in the model:
"levels": [
{
"name":"category",
"label":"Category",
"attributes": ["category"]
},
{
"name":"subcategory",
"label":"Sub-category",
"attributes": ["subcategory"]
},
{
"name":"line_item",
"label":"Line Item",
"attributes": ["line_item"]
}
]
You can see a slight difference between this model description and the previous one: we didn’t just specify
level names and didn’t let cubes to fill-in the defaults. Here we used explicit description of each level.
name
is level identifier, label
is human-readable label of the level that can be
used in end-user applications and attributes
is list of attributes that belong to the level.
The first attribute, if not specified otherwise, is the key attribute of the level.
Other level description attributes are key
and label_attribute
. The
key
specifies attribute name which contains key for the level. Key is an id number, code or
anything that uniquely identifies the dimension level. label_attribute
is name of an attribute
that contains human-readable value that can be displayed in user-interface elements such as tables or
charts.
Preparation
In this how-to we are going to skip all off-topic code, such as data initialization. The full example can
be found in the tutorial sources with suffix
03
.
In short we need:
- data in a database
- logical model (see
model_03.json
) prepared with appropriate mappings
- denormalized view for aggregated browsing (for current simple SQL browser implementation)
Drill-down
Drill-down is an action that will provide more details about data. Drilling down through a dimension
hierarchy will expand next level of the dimension. It can be compared to browsing through your directory
structure.
We create a function that will recursively traverse a dimension hierarchy and will print-out aggregations
(count of records in this example) at the actual browsed location.
Attributes
- cell - cube cell to drill-down
- dimension - dimension to be traversed through all levels
- path - current path of the
dimension
Path is list of dimension points (keys) at each level. It is like file-system path.
def drill_down(cell, dimension, path = []):
Get dimension’s default hierarchy. Cubes supports multiple hierarchies, for example for date you might
have year-month-day or year-quarter-month-day. Most dimensions will have one hierarchy, thought.
hierarchy = dimension.default_hierarchy
Base path is path to the most detailed element, to the leaf of a tree, to the fact. Can we go deeper in
the hierarchy?
if hierarchy.path_is_base(path):
return
Get the next level in the hierarchy. levels_for_path
returns list of levels according to
provided path. When drilldown
is set to True
then one more level is returned.
levels = hierarchy.levels_for_path(path,drilldown=True)
current_level = levels[-1]
We need to know name of the level key attribute which contains a path component. If the model does not
explicitly specify key attribute for the level, then first attribute will be used:
level_key = dimension.attribute_reference(current_level.key)
For prettier display, we get name of attribute which contains label to be displayed
for the current level. If there is no label attribute, then key attribute is used.
level_label = dimension.attribute_reference(current_level.label_attribute)
We do the aggregation of the cell… Think of ls $CELL
command in commandline, where
$CELL
is a directory name. In this function we can think of $CELL
to be same as
current working directory (pwd
)
result = browser.aggregate(cell, drilldown=[dimension])
for record in result.drilldown:
print "%s%s: %d" % (indent, record[level_label], record["record_count"])
...
And now the drill-down magic. First, construct new path by key attribute value appended to the current
path:
drill_path = path[:] + [record[level_key]]
Then get a new cell slice for current path:
drill_down_cell = cell.slice(dimension, drill_path)
And do recursive drill-down:
drill_down(drill_down_cell, dimension, drill_path)
The function looks like this:
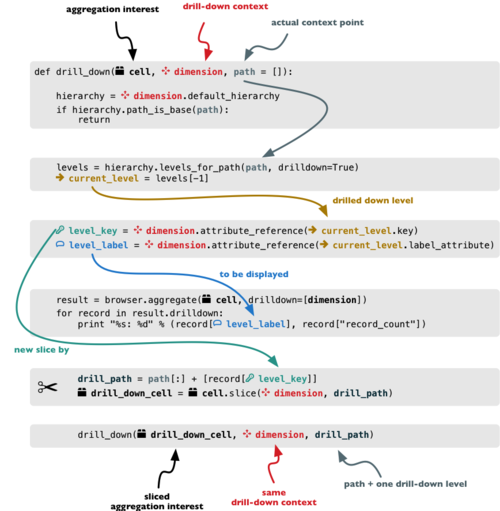
Working function example 03
can be found in the tutorial
sources.
Get the full cube (or any part of the cube you like):
cell = browser.full_cube()
And do the drill-down through the item dimension:
drill_down(cell, cube.dimension("item"))
The output should look like this:
a: 32
da: 8
Borrowings: 2
Client operations: 2
Investments: 2
Other: 2
dfb: 4
Currencies subject to restriction: 2
Unrestricted currencies: 2
i: 2
Trading: 2
lo: 2
Net loans outstanding: 2
nn: 2
Nonnegotiable, nonintrest-bearing demand obligations on account of subscribed capital: 2
oa: 6
Assets under retirement benefit plans: 2
Miscellaneous: 2
Premises and equipment (net): 2
Note that because we have changed our source data, we see level codes instead of level names. We will fix
that later. Now focus on the drill-down.
See that nice hierarchy tree?
Now if you slice the cell through year 2010 and do the exact same drill-down:
cell = cell.slice("year", [2010])
drill_down(cell, cube.dimension("item"))
you will get similar tree, but only for year 2010 (obviously).
Level Labels and Details
Codes and ids are good for machines and programmers, they are short, might follow some scheme, easy to
handle in scripts. Report users have no much use of them, as they look cryptic and have no meaning for the
first sight.
Our source data contains two columns for category and for subcategory: column with code and column with
label for user interfaces. Both columns belong to the same dimension and to the same level. The key column
is used by the analytical system to refer to the dimension point and the label is just decoration.
Levels can have any number of detail attributes. The detail attributes have no analytical meaning and are
just ignored during aggregations. If you want to do analysis based on an attribute, make it a separate
dimension instead.
So now we fix our model by specifying detail attributes for the levels:

The model description is:
"levels": [
{
"name":"category",
"label":"Category",
"label_attribute": "category_label",
"attributes": ["category", "category_label"]
},
{
"name":"subcategory",
"label":"Sub-category",
"label_attribute": "subcategory_label",
"attributes": ["subcategory", "subcategory_label"]
},
{
"name":"line_item",
"label":"Line Item",
"attributes": ["line_item"]
}
]
}
Note the label_attribute
keys. They specify which attribute contains label to be displayed.
Key attribute is by-default the first attribute in the list. If one wants to use some other attribute it
can be specified in key_attribute
.
Because we added two new attributes, we have to add mappings for them:
"mappings": { "item.line_item": "line_item",
"item.subcategory": "subcategory",
"item.subcategory_label": "subcategory_label",
"item.category": "category",
"item.category_label": "category_label"
}
In the example tutorial, which can be found in the Cubes sources under tutorial/
directory,
change the model file from model/model_03.json
to model/model_03-labels.json
and run the code again. Or fix the file as specified above.
Now the result will be:
Assets: 32
Derivative Assets: 8
Borrowings: 2
Client operations: 2
Investments: 2
Other: 2
Due from Banks: 4
Currencies subject to restriction: 2
Unrestricted currencies: 2
Investments: 2
Trading: 2
Loans Outstanding: 2
Net loans outstanding: 2
Nonnegotiable: 2
Nonnegotiable, nonintrest-bearing demand obligations on account of subscribed capital: 2
Other Assets: 6
Assets under retirement benefit plans: 2
Miscellaneous: 2
Premises and equipment (net): 2
Implicit hierarchy
Try to remove the last level line_item from the model file and see what happens. Code still works, but
displays only two levels. What does that mean? If metadata - logical model - is used properly in an
application, then application can handle most of the model changes without any application modifications.
That is, if you add new level or remove a level, there is no need to change your reporting application.
Summary
- hierarchies can have multiple levels
- a hierarchy level is identifier by a key attribute
- a hierarchy level can have multiple detail attributes and there is one special detail attribute: label attribute used for display in user interfaces
Next: slicing and dicing or slicer server, not sure yet.
If you have any questions, suggestions, comments, let me know.