2012-05-12 by Stefan Urbanek
Last time I was talking about joins and
denormalisation in the Star
Browser. This is the last part about the star browser where I will describe the aggregation and what has changed, compared to the old browser.
The Star Browser is new aggregation browser in for the Cubes – lightweight
Python OLAP Framework. Next version v0.9 will be released next week.
Aggregation
sum is not the only aggregation. The new browser allows to have other
aggregate functions as well, such as min, max.
You can specify the aggregations for each measure separately:
{
"name": "amount",
"aggregations": ["sum", "min", "max"]
}
The resulting aggregated attribute name will be constructed from the measure
name and aggregation suffix, for example the mentioned amount will have
three aggregates in the result: amount_sum
, amount_min
and amount_max
.
Source code reference: see StarQueryBuilder.aggregations_for_measure
Aggregation Result
Result of aggregation is a structure containing: summary
- summary for the
aggregated cell, drilldown
- drill down cells, if was desired, and
total_cell_count
- total cells in the drill down, regardless of pagination.
Cell Details
When we are browsing the cube, the cell provides current browsing context. For
aggregations and selections to happen, only keys and some other internal
attributes are necessary. Those can not be presented to the user though. For
example we have geography path (country
, region
) as ['sk', 'ba']
,
however we want to display to the user Slovakia
for the country and
Bratislava
for the region. We need to fetch those values from the data
store. Cell details is basically a human readable description of the current
cell.
For applications where it is possible to store state between aggregation
calls, we can use values from previous aggregations or value listings. Problem
is with web applications - sometimes it is not desirable or possible to store
whole browsing context with all details. This is exact the situation where
fetching cell details explicitly might come handy.
Note: The Original browser added cut information in the summary, which was ok
when only point cuts were used. In other situations the result was undefined
and mostly erroneous.
The cell details are now provided separately by method
AggregationBrowser.cell_details(cell)
which has Slicer HTTP equivalent
/details
or {"query":"detail", ...}
in /report
request. The result is
a list of
For point cuts, the detail is a list of dictionaries for each level. For
example our previously mentioned path ['sk', 'ba']
would have details
described as:
[
{
"geography.country_code": "sk",
"geography.country_name": "Slovakia",
"geography.something_more": "..."
"_key": "sk",
"_label": "Slovakia"
},
{
"geography.region_code": "ba",
"geography.region_name": "Bratislava",
"geography.something_even_more": "...",
"_key": "ba",
"_label": "Bratislava"
}
]
You might have noticed the two redundant keys: _key
and _label
- those
contain values of a level key attribute and level label attribute
respectively. It is there to simplify the use of the details in presentation
layer, such as templates. Take for example doing only one-dimensional
browsing and compare presentation of "breadcrumbs":
labels = [detail["_label"] for detail in cut_details]
Which is equivalent to:
levels = dimension.hierarchy.levels()
labels = []
for i, detail in enumerate(cut_details):
labels.append(detail[level[i].label_attribute.full_name()])
Note that this might change a bit: either full detail will be returned or just
key and label, depending on an option argument (not yet decided).
Pre-aggregation
The Star Browser is being created with SQL pre-aggregation in mind. This is
not possible in the old browser, as it is not flexible enough. It is planned
to be integrated when all basic features are finished.
Proposed access from user's perspective will be through configuration options:
use_preaggregation
, preaggregation_prefix
, preaggregation_schema
and
a method for cube pre-aggregation will be available through the slicer tool.
Summary
The new browser has better internal structure resulting in increased
flexibility for future extensions. It fixes not so good architectural
decisions of the old browser.
New and fixed features:
- direct star/snowflake schema browsing
- improved mappings - more transparent and understandable process
- ability to explicitly specify database schemas
- multiple aggregations
The new backend sources are
here
and the mapper is
here.
To do
To be done in the near future:
- DDL generator for denormalized schema, corresponding logical schema and
physical schema
- explicit list of attributes to be selected (instead of all)
- selection of aggregations per-request (now all specified in model are used)
Links
See also Cubes at github,
Cubes Documentation,
Mailing List
and Submit issues. Also there is an
IRC channel #databrewery on
irc.freenode.net
2012-05-01 by Stefan Urbanek
Last time I was talking about how logical attributes are mapped to the
physical table columns in the
Star Browser. Today I will describe how joins are formed and how
denormalization is going to be used.
The Star Browser is new aggregation browser in for the
Cubes – lightweight Python OLAP Framework.
Star, Snowflake, Master and Detail
Star browser supports a star:
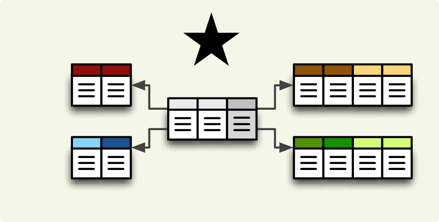
... and snowflake database schema:
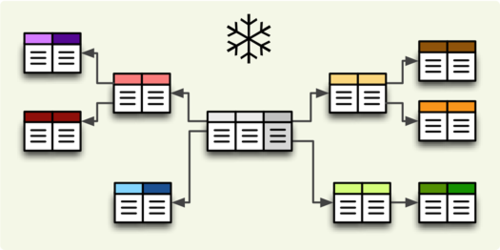
The browser should know how to construct the star/snowflake and that is why
you have to specify the joins of the schema. The join specification is very
simple:
"joins" = [
{ "master": "fact_sales.product_id", "detail": "dim_product.id" }
]
Joins support only single-column keys, therefore you might have to create
surrogate keys for your dimensions.
As in mappings, if you have specific needs for explicitly mentioning database
schema or any other reason where table.column
reference is not enough, you
might write:
"joins" = [
{
"master": "fact_sales.product_id",
"detail": {
"schema": "sales",
"table": "dim_products",
"column": "id"
}
]
What if you need to join same table twice? For example, you have list of
organizations and you want to use it as both: supplier and service consumer.
It can be done by specifying alias in the joins:
"joins" = [
{
"master": "contracts.supplier_id",
"detail": "organisations.id",
"alias": "suppliers"
},
{
"master": "contracts.consumer_id",
"detail": "organisations.id",
"alias": "consumers"
}
]
In the mappings you refer to the table by alias specified in the joins, not by
real table name:
"mappings": {
"supplier.name": "suppliers.org_name",
"consumer.name": "consumers.org_name"
}
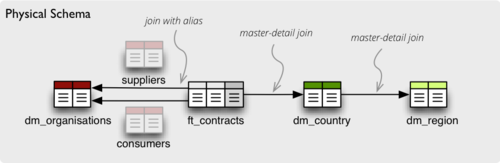
Relevant Joins and Denormalization
The new mapper joins only tables that are relevant for given query. That is,
if you are browsing by only one dimension, say product, then only product
dimension table is joined.
Joins are slow, expensive and the denormalization can be
helpful:
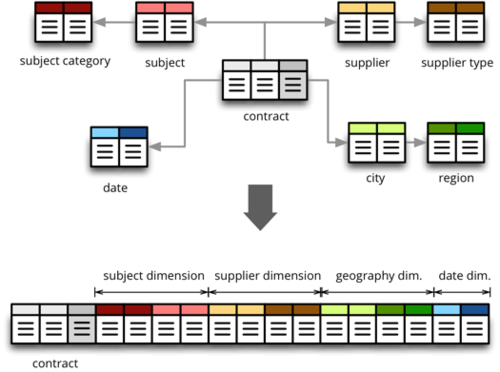
The old browser is based purely on the denormalized view. Despite having a
performance gain, it has several disadvantages. From the
join/performance perspective the major one is, that the denormalization is
required and it is not possible to browse data in a database that was
"read-only". This requirements was also one unnecessary step for beginners,
which can be considered as usability problem.
Current implementation of the Mapper and StarBrowser allows
denormalization to be integrated in a way, that it might be used based on
needs and situation:
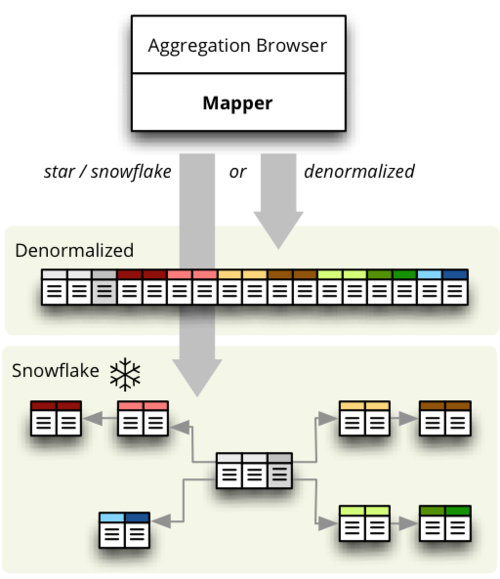
It is not yet there and this is what needs to be done:
- function for denormalization - similar to the old one: will take cube and
view name and will create denormalized view (or a table)
- make mapper accept the view and ignore joins
Goal is not just to slap denormalization in, but to make it a configurable
alternative to default star browsing. From user's perspective, the workflow
will be:
- browse star/snowflake until need for denormalization arises
- configure denormalization and create denormalized view
- browse the denormalized view
The proposed options are: use_denormalization
, denormalized_view_prefix
,
denormalized_view_schema
.
The Star Browser is half-ready for the denormalization, just few changes are
needed in the mapper and maybe query builder. These changes have to be
compatible with another, not-yet-included feature: SQL pre-aggregation.
Conclusion
The new way of joining is very similar to the old one, but has much more
cleaner code and is separated from mappings. Also it is more transparent. New
feature is the ability to specify a database schema. Planned feature to be
integrated is automatic join detection based on foreign keys.
In the next post (the last post in this series) about the new StarBrowser, I am going to
explain aggregation improvements and changes.
Links
Relevant source code is this one (github).
See also Cubes at github,
Cubes Documentation,
Mailing List
and Submit issues. Also there is an
IRC channel #databrewery on
irc.freenode.net
2012-04-30 by Stefan Urbanek
Star Browser is new aggregation browser in for the
Cubes – lightweight Python OLAP Framework.
I am going to talk briefly about current state and why new browser is needed.
Then I will describe in more details the new browser: how mappings work, how
tables are joined. At the end I will mention what will be added soon and what
is planned in the future.
Originally I wanted to write one blog post about this, but it was too long, so
I am going to split it into three:
Why new browser?
Current denormalized
browser
is good, but not good enough. Firstly, it has grown into a spaghetti-like
structure inside and adding new features is quite difficult. Secondly, it is
not immediately clear what is going on inside and not only new users are
getting into troubles. For example the mapping of logical to physical is not
obvious; denormalization is forced to be used, which is good at the end, but
is making OLAP newbies puzzled.
The new browser, called
StarBrowser.
is half-ready and will fix many of the old decisions with better ones.
Mapping
Cubes provides an analyst's view of dimensions and their attributes by hiding
the physical representation of data. One of the most important parts of proper
OLAP on top of the relational database is the mapping of physical attributes
to logical.
First thing that was implemented in the new browser is proper mapping of
logical attributes to physical table columns. For example, take a reference to
an attribute name in a dimension product. What is the column of what table
in which schema that contains the value of this dimension attribute?
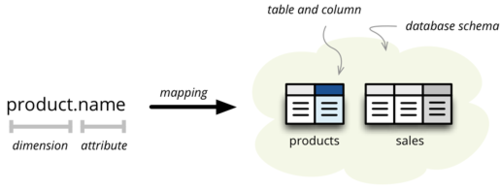
There are two ways how the mapping is being done: implicit and explicit. The
simplest, straightforward and most customizable is the explicit way, where the
actual column reference is provided in the model description:
"mappings": {
"product.name": "dm_products.product_name"
}
If it is in different schema or any part of the reference contains a dot:
"mappings": {
"product.name": {
"schema": "sales",
"table": "dm_products",
"column": "product_name"
}
}
Disadvantage of the explicit way is it's verbosity and the fact that developer
has to write more metadata, obviously.
Both, explicit and implicit mappings have ability to specify default database
schema (if you are using Oracle, PostgreSQL or any other DB which supports
schemas).
The mapping process process is like this:
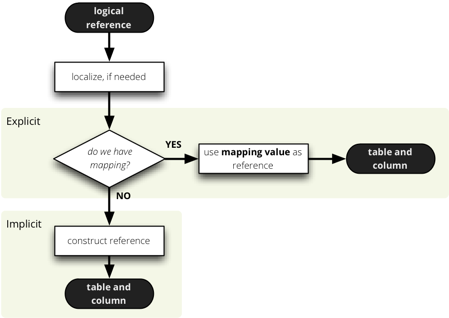
Implicit Mapping
With implicit mapping one can match a database schema with logical model and
does not have to specify additional mapping metadata. Expected structure is
star schema with one table per (denormalized) dimension.
Basic rules:
- fact table should have same name as represented cube
- dimension table should have same name as the represented dimension, for
example:
product
(singular)
- references without dimension name in them are expected to be in the fact
table, for example:
amount
, discount
(see note below for simple flat
dimensions)
- column name should have same name as dimension attribute:
name
, code
,
description
- if attribute is localized, then there should be one column per localization
and should have locale suffix:
description_en
, description_sk
,
description_fr
(see below for more information)
This means, that by default product.name
is mapped to the table product
and column name
. Measure amount
is mapped to the table sales
and column
amount
What about dimensions that have only one attribute, like one would not have a
full date but just a year
? In this case it is kept in the fact table without
need of separate dimension table. The attribute is treated in by the same rule
as measure and is referenced by simple year
. This is applied to all
dimensions that have only one attribute (representing key as well). This
dimension is referred to as flat and without details.
Note for advanced users: this behavior can be disabled by setting
simplify_dimension_references
to False
in the mapper. In that case you
will have to have separate table for the dimension attribute and you will have
to reference the attribute by full name. This might be useful when you know
that your dimension will be more detailed.
Localization
Despite localization taking place first in the mapping process, we talk about
it at the end, as it might be not so commonly used feature. From physical
point of view, the data localization is very trivial and requires language
denormalization - that means that each language has to have its own column for
each attribute.
In the logical model, some of the attributes may contain list of locales that
are provided for the attribute. For example product category can be in
English, Slovak or German. It is specified in the model like this:
attributes = [{
"name" = "category",
"locales" = [en, sk, de],
}]
During the mapping process, localized logical reference is created first:
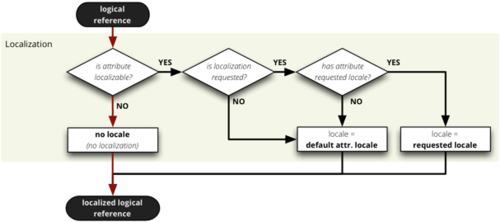
In short: if attribute is localizable and locale is requested, then locale
suffix is added. If no such localization exists then default locale is used.
Nothing happens to non-localizable attributes.
For such attribute, three columns should exist in the physical model. There
are two ways how the columns should be named. They should have attribute name
with locale suffix such as category_sk
and category_en
(underscore
because it is more common in table column names), if implicit mapping is used.
You can name the columns as you like, but you have to provide explicit mapping
in the mapping dictionary. The key for the localized logical attribute should
have .locale
suffix, such as product.category.sk
for Slovak version of
category attribute of dimension product. Here the dot is used because dots
separate logical reference parts.
Customization of the Implicit
The implicit mapping process has a little bit of customization as well:
- dimension table prefix: you can specify what prefix will be used for all
dimension tables. For example if the prefix is
dim_
and attribute is
product.name
then the table is going to be dim_product
.
- fact table prefix: used for constructing fact table name from cube name.
Example: having prefix
ft_
all fact attributes of cube sales
are going
to be looked up in table ft_sales
- fact table name: one can explicitly specify fact table name for each cube
separately
The Big Picture
Here is the whole mapping schema, after localization:
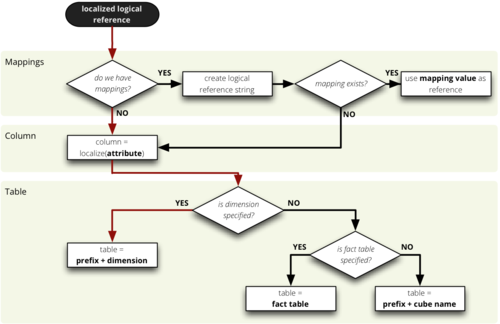
Links
The commented mapper source is
here.
2012-04-29 by Stefan Urbanek
I've been working on a new SQL backend for cubes called StarBrowser. Besides
new features and fixes, it is going to be more polished and maintainable.
Current Backend Comparison
In the following table you can see comparison of backends (or rather
aggregation browsers). Current backend is sql.browser
which reqiures
denormalized table as a source. Future preferred backend will be sql.star
.
Document link at Google Docs.
Star Browser state
More detailed description with schemas and description of what is happening
behind will be published once the browser will be useable in most of the
important features (that is, no sooner than drill-down is implemented). Here
is a peek to the new browser features.
- separated attribute mapper - doing the logical-to-physical mapping. or in
other words: knows what column in which table represents what dimension
attribute or a measure
- more intelligent join building - uses only joins that are relevant to the
retrieved attributes, does not join the whole star/snowflake if not necessary
- allows tables to be stored in different database schemas (previously
everything had to be in one schema)
There is still some work to be done, including drill-down and ordering
of results.
You can try limited feature set of the browser by using sql.star
backend
name. Do not expect much at this time, however if you find a bug, I would be
glad if report it through github
issues. The source is in the
cubes/backends/sql/star.py
and cubes/backends/sql/common.py
(or
here).
New and improved
Here is a list of features you can expect (not yet fully implemented, if at
all started):
- more SQL aggregation types and way to specify what aggregations
should be used by-default for each measure
- DDL schema generator for: denormalized table, logical model - star schema,
physical model
- model tester - tests whether all attributes and joins are valid in the
physical model
Also the new implementation of star browser will allow easier integration of
pre-aggregated store (planned) and various other optimisations.
2012-04-13 by Stefan Urbanek
How to build and run a data analysis stream? Why streams? I am going to talk about
how to use brewery from command line and from Python scripts.
Brewery is a Python framework and a way of analysing and auditing data. Basic
principle is flow of structured data through processing and analysing nodes.
This architecture allows more transparent, understandable and maintainable
data streaming process.
You might want to use brewery when you:
- want to learn more about data
- encounter unknown datasets and/or you do not know what you have in your
datasets
- do not know exactly how to process your data and you want to play-around
without getting lost
- want to create alternative analysis paths and compare them
- measure data quality and feed data quality results into the data processing
process
There are many approaches and ways how to the data analysis. Brewery brings a certain workflow to the analyst:
- examine data
- prototype a stream (can use data sampling, not to overheat the machine)
- see results and refine stream, create alternatives (at the same time)
- repeat 3. until satisfied
Brewery makes the steps 2. and 3. easy - quick prototyping, alternative
branching, comparison. Tries to keep the analysts workflow clean and understandable.
Building and Running a Stream
There are two ways to create a stream: programmatic in Python and command-line
without Python knowledge requirement. Both ways have two alternatives: quick
and simple, but with limited feature set. And the other is full-featured but
is more verbose.
The two programmatic alternatives to create a stream are: basic construction
and "HOM" or forking construction. The two command line ways to run a
stream: run and pipe. We are now going to look closer at them.
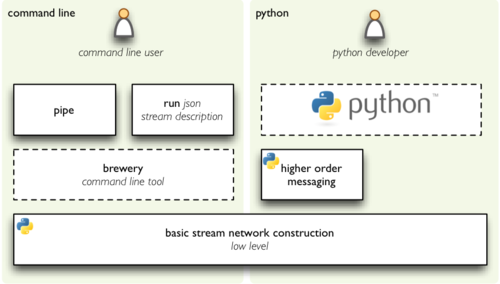
Note regarding Zen of Python: this does not go against "There should be one –
and preferably only one – obvious way to do it." There is only one way: the
raw construction. The others are higher level ways or ways in different
environments.
In our examples below we are going to demonstrate simple linear (no branching)
stream that reads a CSV file, performs very basic audit and "pretty prints"
out the result. The stream looks like this:
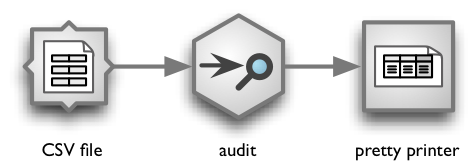
Command line
Brewery comes with a command line utility brewery
which can run streams
without needing to write a single line of python code. Again there are two
ways of stream description: json-based and plain linear pipe.
The simple usage is with brewery pipe
command:
brewery pipe csv_source resource=data.csv audit pretty_printer
The pipe
command expects list of nodes and attribute=value
pairs for node
configuration. If there is no source pipe specified, CSV on standard input is
used. If there is no target pipe, CSV on standard output is assumed:
cat data.csv | brewery pipe audit
The actual stream with implicit nodes is:
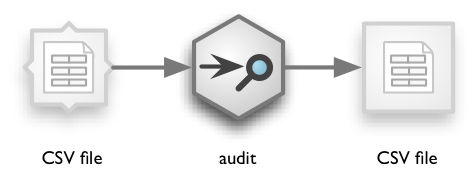
The json
way is more verbose but is full-featured: you can create complex
processing streams with many branches. stream.json
:
{
"nodes": {
"source": { "type":"csv_source", "resource": "data.csv" },
"audit": { "type":"audit" },
"target": { "type":"pretty_printer" }
},
"connections": [
["source", "audit"],
["audit", "target"]
]
}
And run:
$ brewery run stream.json
To list all available nodes do:
To get more information about a node, run brewery nodes
:
$ brewery nodes string_strip
Note that data streaming from command line is more limited than the python
way. You might not get access to nodes and node features that require python
language, such as python storage type nodes or functions.
Higher order messaging
Preferred programming way of creating streams is through higher order
messaging (HOM), which is, in this case, just fancy name for pretending doing
something while in fact we are preparing the stream.
This way of creating a stream is more readable and maintainable. It is easier
to insert nodes in the stream and create forks while not losing picture of the
stream. Might be not suitable for very complex streams though. Here is an
example:
b = brewery.create_builder()
b.csv_source("data.csv")
b.audit()
b.pretty_printer()
When this piece of code is executed, nothing actually happens to the data
stream. The stream is just being prepared and you can run it anytime:
b.stream.run()
What actually happens? The builder b
is somehow empty object that accepts
almost anything and then tries to find a node that corresponds to the method
called. Node is instantiated, added to the stream and connected to the
previous node.
You can also create branched stream:
b = brewery.create_builder()
b.csv_source("data.csv")
b.audit()
f = b.fork()
f.csv_target("audit.csv")
b.pretty_printer()
Basic Construction
This is the lowest level way of creating the stream and allows full
customisation and control of the stream. In the basic construction method
the programmer prepares all node instance objects and connects them
explicitly, node-by-node. Might be a too verbose, however it is to be used by
applications that are constructing streams either using an user interface or
from some stream descriptions. All other methods are using this one.
from brewery import Stream
from brewery.nodes import CSVSourceNode, AuditNode, PrettyPrinterNode
stream = Stream()
# Create pre-configured node instances
src = CSVSourceNode("data.csv")
stream.add(src)
audit = AuditNode()
stream.add(audit)
printer = PrettyPrinterNode()
stream.add(printer)
# Connect nodes: source -> target
stream.connect(src, audit)
stream.connect(audit, printer)
stream.run()
It is possible to pass nodes as dictionary and connections as list of tuples
(source, target):
stream = Stream(nodes, connections)
Future plans
What would be lovely to have in brewery?
Probing and data quality indicators – tools for simple data probing and
easy way of creating data quality indicators. Will allow something like
"test-driven-development" but for data. This is the next step.
Stream optimisation – merge multiple nodes into single processing unit
before running the stream. Might be done in near future.
Backend-based nodes and related data transfer between backend nodes – For
example, two SQL nodes might pass data through a database table instead of
built-in data pipe or two numpy/scipy-based nodes might use numpy/scipy
structure to pass data to avoid unnecessary streaming. Not very soon, but
foreseeable future.
Stream compilation – compile a stream to an optimised script. Not too
soon, but like to have that one.
Last, but not least: Currently there is little performance cost because of the
nature of brewery implementation. This penalty will be explained in another
blog post, however to make long story short, it has to do with threads, Python
GIL and non-optimalized stream graph. There is no future prediction for this
one, as it might be included step-by-step. Also some Python 3 features look
promising, such as yield from
in Python 3.3 (PEP 308).
Links