2012-04-04 by Stefan Urbanek
I'm glad to announce new release of Brewery – stream based data auditing and analysis framework for Python.
There are quite a few updates, to mention the notable ones:
- new
brewery
runner with commands run
and graph
- new nodes: pretty printer node (for your terminal pleasure), generator
function node
- many CSV updates and fixes
Added several simple how-to
examples, such as:
aggregation of remote CSV, basic audit of a CSV, how to use a generator
function. Feedback and questions are welcome. I'll help you.
Note that there are couple changes that break compatibility, however they can
be updated very easily. I apologize for the inconvenience, but until 1.0 the
changes might happen more frequently. On the other hand, I will try to make
them as painless as possible.
Full listing of news, changes and fixes is below.
Version 0.8
News
- Changed license to MIT
- Created new brewery runner commands: 'run' and 'graph':
- 'brewery run stream.json' will execute the stream
- 'brewery graph stream.json' will generate graphviz data
- Nodes: Added pretty printer node - textual output as a formatted table
- Nodes: Added source node for a generator function
- Nodes: added analytical type to derive field node
- Preliminary implementation of data probes (just concept, API not decided yet
for 100%)
- CSV: added empty_as_null option to read empty strings as Null values
-
Nodes can be configured with node.configure(dictionary, protected). If
'protected' is True, then protected attributes (specified in node info) can
not be set with this method.
-
added node identifier to the node reference doc
-
added create_logger
-
added experimental retype feature (works for CSV only at the moment)
- Mongo Backend - better handling of record iteration
Changes
- CSV: resource is now explicitly named argument in CSV*Node
- CSV: convert fields according to field storage type (instead of all-strings)
- Removed fields getter/setter (now implementation is totally up to stream
subclass)
- AggregateNode: rename
aggregates
to measures
, added measures
as
public node attribute
- moved errors to brewery.common
- removed
field_name()
, now str(field) should be used
- use named blogger 'brewery' instead of the global one
- better debug-log labels for nodes (node type identifier + python object ID)
WARNING: Compatibility break:
- depreciate
__node_info__
and use plain node_info
instead
Stream.update()
now takes nodes and connections as two separate arguments
Fixes
- added SQLSourceNode, added option to keep ifelds instead of dropping them in
FieldMap and FieldMapNode (patch by laurentvasseur @ bitbucket)
- better traceback handling on node failure (now actually the traceback is
displayed)
- return list of field names as string representation of FieldList
- CSV: fixed output of zero numeric value in CSV (was empty string)
Links
- github sources: https://github.com/Stiivi/brewery
- Documentation: http://packages.python.org/brewery/
- Mailing List: http://groups.google.com/group/databrewery/
- Submit issues here: https://github.com/Stiivi/brewery/issues
- IRC channel: #databrewery on irc.freenode.net
If you have any questions, comments, requests, do not hesitate to ask.
2012-03-09 by Stefan Urbanek
Another minor release of Cubes - Light Weight Python OLAP framework is out. Main change is that backend is no longer hard-wired in the Slicer server and can be selected through configuration file.
There were lots of documentation changes, for example the reference was separated from the rest of docs. Hello World! example was added.
The news, changes and fixes are:
New Features
- Started writing StarBrowser - another SQL aggregation browser with different
approach (see code/docs)
Slicer Server:
- added configuration option
modules
under [server]
to load additional
modules
- added ability to specify backend module
- backend configuration is in [backend] by default, for SQL it stays in [db]
- added server config option for default
prettyprint
value (useful for
demontration purposes)
Documentation:
- Changed license to MIT + small addition. Please refer to the LICENSE file.
- Updated documentation - added missing parts, made reference more readable,
moved class and function reference docs from descriptive part to reference
(API) part.
- added backend documentation
- Added "Hello World!" example
Changed Features
- removed default SQL backend from the server
- moved worskpace creation into the backend module
Fixes
- Fixed create_view to handle not materialized properly (thanks to deytao)
- Slicer tool header now contains #!/usr/bin/env python
Links
- github sources: https://github.com/Stiivi/cubes
- Documentation: http://packages.python.org/cubes/
- Mailing List: http://groups.google.com/group/cubes-discuss
- Submit issues here: https://github.com/Stiivi/cubes/issues
- IRC channel: #databrewery on irc.freenode.net
If you have any questions, comments, requests, do not hesitate to ask.
2012-02-23 by Stefan Urbanek
Cubes - The Lightweight Python OLAP Framework is now licensed under the MIT license with small addition. The full license is as follows:
Copyright (c) 2011-2012 Stefan Urbanek, see AUTHORS for more details
Permission is hereby granted, free of charge, to any person obtaining a copy of this software and
associated documentation files (the "Software"), to deal in the Software without restriction, including
without limitation the rights to use, copy, modify, merge, publish, distribute, sublicense, and/or sell
copies of the Software, and to permit persons to whom the Software is furnished to do so, subject to
the following conditions:
-
The above copyright notice and this permission notice shall be included in all copies or substantial
portions of the Software.
-
If your version of the Software supports interaction with it remotely through a computer network, the
above copyright notice and this permission notice shall be accessible to all users.
THE SOFTWARE IS PROVIDED "AS IS", WITHOUT WARRANTY OF ANY KIND, EXPRESS OR IMPLIED, INCLUDING BUT NOT
LIMITED TO THE WARRANTIES OF MERCHANTABILITY, FITNESS FOR A PARTICULAR PURPOSE AND NONINFRINGEMENT. IN
NO EVENT SHALL THE AUTHORS OR COPYRIGHT HOLDERS BE LIABLE FOR ANY CLAIM, DAMAGES OR OTHER LIABILITY,
WHETHER IN AN ACTION OF CONTRACT, TORT OR OTHERWISE, ARISING FROM, OUT OF OR IN CONNECTION WITH THE
SOFTWARE OR THE USE OR OTHER DEALINGS IN THE SOFTWARE.
The addition says, that if you use it as part of software as a service (SaaS) you have to provide the copyright notice in an about, legal info, credits or some similar kind of page or info box. That's all.
May it be like that? :-)
Updated Cubes sources are here, as usual.
Enjoy.
2012-02-21 by Stefan Urbanek
What is inside the Cubes Python OLAP Framework? Here is a brief overview of the core modules, their purpose and functionality.
The lightweight framework Cubes is composed of four public modules:
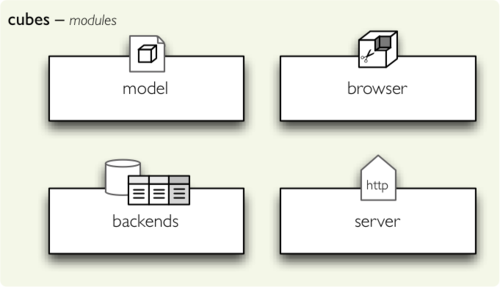
- model - Description of data (metadata): dimensions, hierarchies, attributes, labels, localizations.
- browser - Aggregation browsing, slicing-and-dicing, drill-down.
- backends - Actual aggregation implementation and utility functions.
- server - WSGI HTTP server for Cubes
Model
Logical model describes the data from user’s or analyst’s perspective: data how they are being measured, aggregated and reported. Model is independent of physical implementation of data. This physical independence makes it easier to focus on data instead on ways of how to get the data in understandable form.
Cubes model is described by:
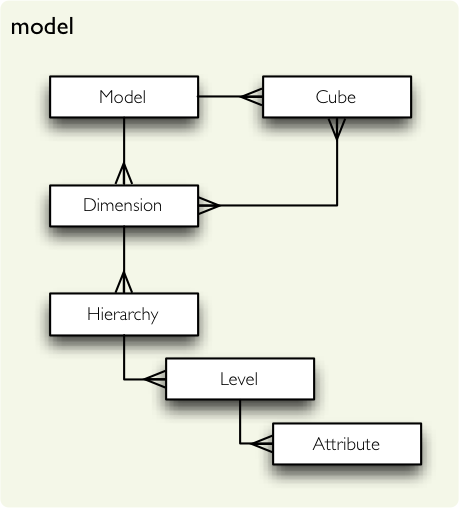
- model object (doc)
- list of cubes
- dimensions of cubes (they are shared with all cubes within model) (doc) (doc)
- hierarchies (doc) and hierarchy levels (doc) of dimensions (such as category-subcategory, country-region-city)
- optional mappings from logical model to the physical model (doc)
- optional join specifications for star schemas, used by the SQL denormalizing backend (doc)
There is a utility function provided for loading the model from a JSON file: load_model
.
The model module object are capable of being localized (see Model Localization for more information). The cubes provides localization at the metadata level (the model) and functionality to have localization at the data level.
See also: Model Documentation
Browser
Core of the Cubes analytics functionality is the aggregation browser. The browser
module contains utility classes and functions for the browser to work.
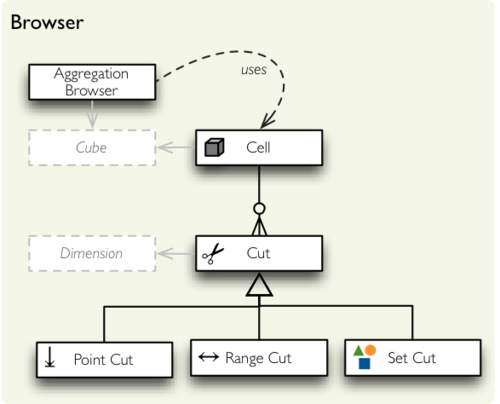
The module components are:
- Cell – specification of the portion of the cube to be explored, sliced or drilled down. Each cell is specified by a set of cuts. A cell without any cuts represents whole cube.
- Cut – definition where the cell is going to be sliced through single dimension. There are three types of cuts: point, range and set.
The types of cuts:
- Point Cut – Defines one single point on a dimension where the cube is going to be sliced. The point might be at any level of hierarchy. The point is specified by "path". Examples of point cut:
[2010]
for year level of Date dimension, [2010,1,7]
for full date point.
- Range Cut – Defines two points (dimension paths) on a sortable dimension between whose the cell is going to be sliced from cube.
- Set Cut – Defines list of multiple points (dimension paths) which are going to be included in the sliced cell.
Example of point cut effect:
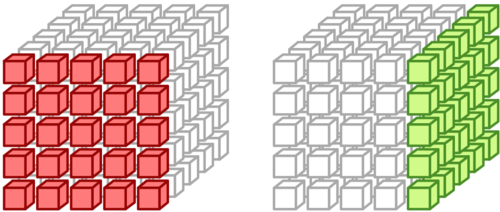
The module provides couple utility functions:
path_from_string
- construct a dimension path (point) from a string
string_from_path
- get a string representation of a dimension path (point)
string_from_cuts
and cuts_from_string
are for conversion between string and list of cuts. (Currently only list of point cuts are supported in the string representation)
The aggregation browser can:
- aggregate a cell (
aggregate(cell)
)
- drill-down through multiple dimensions and aggregate (
aggregate(cell, drilldown="date")
)
- get all detailed facts within the cell (
facts(cell)
)
- get single fact (
fact(id)
)
There is convenience function report(cell, report)
that can be implemented by backend in more efficient way to get multiple aggregation queries in single call.
More about aggregated browsing can be found in the Cubes documentation.
Backends
Actual aggregation is provided by the backends. The backend should implement aggregation browser interface.
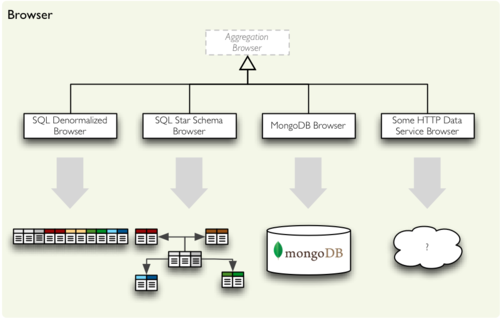
Cubes comes with built-in ROLAP backend which uses SQL database through SQLAlchemy. The backend has two major components:
- aggregation browser that works on single denormalized view or a table
- SQL denormalizer helper class that converts star schema into a denormalized view or table (kind of materialisation).
There was an attempt to write a Mongo DB backend, but it does not work any more, it is included in the sources only as reminder, that there should be a mongo backend sometime in the future.
Anyone can write a backend. If you are interested, drop me a line.
Server
Cubes comes with Slicer - a WSGI HTTP OLAP server with API for most of the cubes framework functionality. The server is based on the Werkzeug framework.
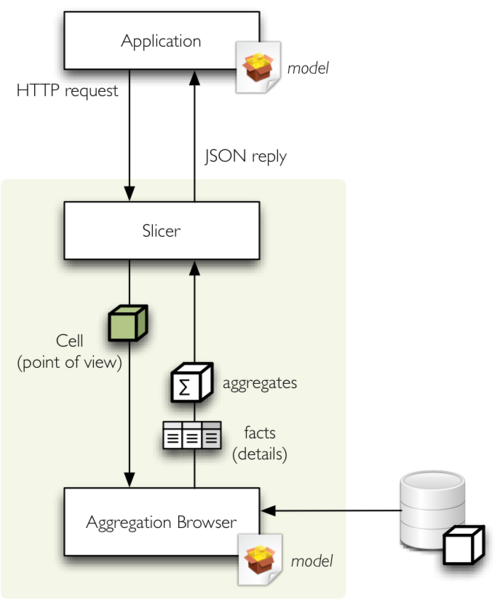
Intended use of the slicer is basically as follows:
- application prepares the cell to be aggregated, drilled, listed... The cell might be whole cube.
- HTTP request is sent to the server
- the server uses appropriate aggregation browser backend (note that currently there is only one: SQL denormalized) to compute the request
- Slicer returns a JSON reply to the application
For more information, please refer to the Cubes Slicer server documentation.
One more thing...
There are plenty things to get improved, of course. Current focus is not on performance, but on achieving simple usability.
The Cubes sources can be found on Github: https://github.com/stiivi/cubes . There is also a IRC channel #databrewery on irc.freenode.net (I try to be there during late evening CET). Issues can be reported on the github project page.
If you have any questions, suggestions, recommendations, just let me know.
HackerNews Thread
2012-02-14 by Stefan Urbanek
I've opened an IRC channel on irc.freenode.net for Data Brewery and Cubes: #databrewery. I will be there mostly during CET daytime/late evening time. Check it out.