2013-04-08 by Stefan Urbanek
Data Brewery home page was redesigned. I would like to thank Andrej Sykora who did a great job with the new look and migration of the old blog posts.
Why?
The main reason for redesign was providing more content for each project. Another one was to have it designed in a way that future projects can be easily added – by having one subdomain for each project.
Important: Blog Moving
The Data Brewery blog is moving away from Tumblr. New blog posts will be generated using Pelican to static pages. The base URL will stay the same: blog.databrewery.org.
The old blog URLs are being redirected to the new URLs. There are still few blog posts that need to be migrated, but we hope to have these finished soon.
If you are following the blog with a feeds reader, here is a link to the new feed.
2013-02-20 by Stefan Urbanek
After few months and gloomy winter nights, here is a humble update of the
Cubes light weight analytical framework. No major feature additions nor
changes this time, except some usability tweaks and fixes.
Documentation was updated to contain relational database patterns for SQL
backend. See the schemas, models and illustrations in the official
documentation.
Also improvements in cross-referencing various documentation parts through
see-also for having relevant information at-hand.
Thanks and credits for support and patches goes to:
- Jose Juan Montes (@jjmontesl)
- Andrew Zeneski
- Reinier Reisy Quevedo Batista (@rquevedo)
Summary
- many improvements in handling multiple hierarchies
- more support of multiple hierarchies in the slicer server either as
parameter or with syntax
dimension@hierarchy
:
- dimension values:
GET /dimension/date?hierarchy=dqmy
- cut: get first quarter of 2012
?cut=date@dqmy:2012,1
- drill-down on hierarchy with week on implicit (next) level:
?drilldown=date@ywd
- drill-down on hierarchy with week with exlpicitly specified week level:
?drilldown=date@ywd:week
- order and order attribute can now be specified for a Level
- optional safe column aliases (see docs for more info) for databases that
have non-standard requirements for column labels even when quoted
New Features
- added
order
to Level object - can be asc
, desc
or None for unspecified
order (will be ignored)
- added
order_attribute
to Level object - specifies attribute to be used for
ordering according to order
. If not specified, then first attribute is
going to be used.
- added hierarchy argument to
AggregationResult.table_rows()
str(cube)
returns cube name, useful in functions that can accept both cube
name and cube object
- added cross table formatter and its HTML variant
GET /dimension
accepts hierarchy parameter
- added
create_workspace_from_config()
to simplify workspace creation
directly from slicer.ini file (this method might be slightly changed in the
future)
to_dict()
method of model objects now has a flag create_label
which
provides label attribute derived from the object's name, if label is missing
- Issue #95: Allow charset to be specified in Content-Type header
SQL:
- added option to SQL workspace/browser
safe_labels
to use safe column
labels for databases that do not support characters like .
in column names
even when quoted (advanced feature, does not work with denormalization)
- browser accepts include_cell_count and include_summary arguments to
optionally disable/enable inclusion of respective results in the aggregation
result object
- added implicit ordering by levels to aggregate and dimension values methods
(for list of facts it is not yet decided how this should work)
- Issue #97: partially implemented sort_key, available in
aggregate()
and
values()
methods
Server:
- added comma separator for
order=
parameter
- reflected multiple search backend support in slicer server
Other:
- added vim syntax highlighting goodie
Changes
- AggregationResult.cross_table is depreciated, use cross table formatter
instead
load_model()
loads and applies translations
- slicer server uses new localization methods (removed localization code from
slicer)
- workspace context provides proper list of locales and new key 'translations'
- added base class Workspace which backends should subclass; backends should
use workspace.localized_model(locale)
create_model()
accepts list of translations
Fixes
- browser.set_locale() now correctly changes browser's locale
- Issue #97: Dimension values call cartesians when cutting by a different
dimension
- Issue #99: Dimension "template" does not copy hierarchies
Links
Sources can be found on github.
Read the documentation.
Join the Google Group for
discussion, problem solving and announcements.
Submit issues and suggestions on github
IRC channel #databrewery on irc.freenode.net
If you have any questions, comments, requests, do not hesitate to ask.
2012-12-09 by Stefan Urbanek
Quick Summary:
- multiple hierarchies:
- Python:
cut = PointCut("date", [2010,15], hierarchy='ywd')
(docs)
- Server:
GET /aggregate?cut=date@ywd:2010,15
(see docs - look for aggregate
documentation)
- Server drilldown:
GET /aggregate?drilldown=date@ywd:week
- added result formatters (experimental! API might change)
- added pre-aggregations (experimental!)
New Features
- added support for multiple hierarchies
- added
dimension_schema
option to star browser – use this when you have
all dimensions grouped in a separate schema than fact table
- added
HierarchyError
- used for example when drilling down deeper than
possible within that hierarchy
- added result formatters: simple_html_table, simple_data_table, text_table
- added create_formatter(formatter_type, options ...)
AggregationResult.levels
is a new dictionary containing levels that the
result was drilled down to. Keys are dimension names, values are levels.
AggregationResult.table_rows()
output has a new variable is_base
to denote
whether the row is base or not in regard to table_rows dimension.
-
added create_server(config_path)
to simplify wsgi script
-
added aggregates: avg, stddev and variance (works only in databases that
support those aggregations, such as PostgreSQL)
-
added preliminary implemenation of pre-aggregation to sql worskspace:
create_conformed_rollup()
create_conformed_rollups()
create_cube_aggregate()
Server:
- multiple drilldowns can be specified in single argument:
drilldown=date,product
- there can be multiple
cut
arguments that will be appended into single cell
- added requests:
GET /cubes
and GET /cube/NAME/dimensions
Changes
- Important: Changed string representation of a set cut: now using
semicolon ';' as a separator instead of a plus symbol '+'
- aggregation browser subclasses should now fill result's
levels
variable
with coalesced_drilldown()
output for requested drill-down levels.
- Moved coalesce_drilldown() from star browser to cubes.browser module to be
reusable by other browsers. Method might be renamed in the future.
- if there is only one level (default) in a dimension, it will have same label
as the owning dimension
- hierarchy definition errors now raise ModelError instead of generic
exception
Fixes
- order of joins is preserved
- fixed ordering bug
- fixed bug in generating conditions from range cuts
AggregationResult.table_rows
now works when there is no point cut
- get correct reference in
table_rows
– now works when simple denormalized
table is used
- raise model exception when a table is missing due to missing join
- search in slicer updated for latest changes
- fixed bug that prevented using cells with attributes in aliased joined
tables
Links
Sources can be found on github.
Read the documentation.
Join the Google Group for discussion, problem solving and announcements.
Submit issues and suggestions on github
IRC channel #databrewery on irc.freenode.net
If you have any questions, comments, requests, do not hesitate to ask.
2012-10-05 by Stefan Urbanek
After a while, here is an update to Cubes - Python Lightweight OLAP framework for multidimensional modeling. There are some changes included that were mentioned in the EruoPython talk such as table_rows
and cross_table
.
I recommend to look at updated examples in the Github repository. The Flask example is now "real" example instead of "sandbox" example and you can see how to generate a simple table for dimension hierarchy browsing.
There is also a more complex example with star-like schema dataset in the cubes-examples github repository. Follow the instructions in README files how to make it running.
There are some backward incompatible changes in this release – until 1.0 the "point" releases will contain this kind of changes, as it is still evolving. You can find more information below.
Quick Summary
- Way how model is constructed has changed. Designated methods are
create_model()
or load_model()
- Dimension defition can have a "template". For example:
{
"name": "contract_date",
"template": "date"
}
- added
table_rows()
and cross_table()
to aggregation result for more convenient table creation. The table_rows
takes care of providing appropriate dimension key and label for browsed level.
- added
simple_model(cube_name, dimension_names, measures)
Incompatibilities: use create_model()
instead of Model(**dict)
, if you
were using just load_model()
, you are fine.
New Features
- To address issue #8
create_model(dict)
was added as replacement for Model(**dict)
. Model()
from
now on will expect correctly constructed model objects. create_model()
will
be able to handle various simplifications and defaults during the
construction process.
- added
info
attribute to all model objects. It can be used to store custom,
application or front-end specific information
- preliminary implementation of
cross_table()
(interface might be changed)
AggregationResult.table_rows()
- new method that iterates through
drill-down rows and returns a tuple with key, label, path, and rest of the
fields.
- dimension in model description can specify another template dimension – all
properties from the template will be inherited in the new dimension. All
dimension properties specified in the new dimension completely override the
template specification
- added
point_cut_for_dimension
- added
simple_model(cube_name, dimensions, measures)
– creates a single-cube
model with flat dimensions from a list of dimension names and measures from
a list of measure names. For example:
model = simple_model("contracts", ["year","contractor", "type"], ["amount"])
Slicer Server:
/cell
– return cell details (replaces /details
)
Changes
- creation of a model from dictionary through
Model(dict)
is depreciated, use
create_model(dict)
instead. All initialization code will be moved there.
Depreciation warnings were added. Old functionality retained for the time
being. (important)
- Replaced
Attribute.full_name()
with Attribute.ref()
- Removed
Dimension.attribute_reference()
as same can be achieved with
dim(attr).ref()
AggregationResult.drilldown
renamed to AggregationResults.cells
(important)
Planned Changes:
str(Attribute)
will return ref() instead of attribute name as it is more
useful
Fixes
- order of dimensions is now preserved in the Model
Links
Sources can be found on github.
Read the documentation.
Join the Google Group for discussion, problem solving and announcements.
Submit issues and suggestions on github
IRC channel #databrewery on irc.freenode.net
If you have any questions, comments, requests, do not hesitate to ask.
2012-08-14 by Stefan Urbanek
What is the data type of 10? Depends on who you are, what are you going to do with it. I would expect my software friends to say that it is an "integer". Why this information might not be sufficient or not relevant? How analysts see the data?
Storage Data Type
If we say "data type", engineers would name types they know from typed programming languages: small integer, double precision float, character. This data type comes from how the data are stored in memory. The type specifies what operations can be done with the data stored at that particuliar place and how much memory is taken. To add two integers on an Intel processor there is an instruction called ADD
, to add two floats there is a different instruction called FADD
(Dear kids: this used to be on a separate chip in PCs!). To add an integer with an float, there has to be a conversion done. Database people would say decimal
, date
or string
. Same as with memory data types, each type has it's allowed set of operations and size it takes in the database. They both are of one kinds of data types: storage data types.
Storage data type, as the name suggests, is used by software (compiler, database system) to know how much memory it takes to store the value of that type and to select appropriate operations (or operation variants).
Concrete vs. Generic
The number of storage data types and their differentiation is exhausting. To name a few:
- C language has more than 25 concrete numeric types and differentiates by floatness, size and sign flag
- PostgreSQL has 9 numeric types, differentiates by size and floatness
- NumPy differentiates not only by size and sign, but also by byte order
Do I need all taht information about data type when working with data? In most cases I don't, it is information for machine, not for me as data analyst/scientist. There are cases when knowing about data types might be handy, like optimisation (for memory consumption for example) or error prevention (of some kind) by type checking in typed languages.
For simplification, some tools use generic data types and hide the concrete storage type: integer
, float
(or real
), string
, ... No storage size, no byte order. Those are low level details.
For reading the data, no input from user is required, as short int
is integer and double
is real. Problem with generic data types is that there might be multiple options how to store a generic integer
.
Analytical Data Types
When doing data analysis I think about variable values and what I can do with them. In data analysis adding two integers or two floats is the same. It is just a + b
. There is only one kind of addition: +
(remember the ADD
and FADD
?). However, there might be numbers that adding them together will have no meaning, like adding two invoice numbers or years together.
To specify how the values should be treated during data analysis, there is another kind of data type: analytical data type or also called variable types. They are:
- Set (or Nominal Variable)
- Values represent categories, like colors or contract. types. Fields of
this type might be numbers which represent for example group numbers, but have
no mathematical interpretation. For example addition of years 1989 and 2012
has no meaning.
- Ordered Set (or Ordinal Variable)
- Similar to set field type, but values can be ordered in a meaningful order.
- Flag (or Binary)
- Special case of set type where values can be one of two types, such as 1 or 0, ‘yes’ or ‘no’, ‘true’ or ‘false’.
- Discrete
- Set of integers - values can be ordered and one can perform arithmetic operations on them, such as: 1 apple + 2 apples = 3 apples.
- Range
- Numerical value, such as financial amount, temperature
The analytical data types are disstinct from storage data types. Take for example just an integer: it can be from a set without any arithmetic operations (ID, year), can be a discrete number (count of something), a flag with binary values of 40
and 50
. Integer as a set can be ordered as set of product sizes or unordered as kind of IDs or category numbers where categories are ordered by their names rather.
In addition to the mentioned data types, it is sometimes useful to specify that the tool or algorithm should just ignore a field/column/variable. For that purpose typeless analytical data type might be used.
Here is an example of storage and analytical data types:
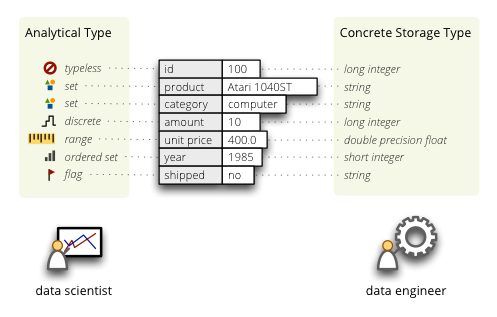
The idea behind analytical data types is described for example in nice introductory data mining book [1] or also in [2]. [1] differentiates measures as interval-scaled variables and ratio-scaled variables. Interesting that [2] describes the "set", which they call "categorical variable" as "generalization of the binary in that it can take one more than two states", not the other way around.
[1] Max Bramer: Principles of Datamining, Springer Verlag London United 2007, p12.
[2] Jaiwen Wan and Micheline Kamber: Data Mining - concepts and techniques, Elsevier 2006, p392.
Keep the metadata with you
As data are passed through algorithms, blocks of processing code, data types (along with other relevant metadata) should be passed with them. Data types can be in some cases guessed from data stream or explicitly expressed by a user, sometimes they can be reflected (like in a database). It is good to keep them, even if sometimes it is not possible to maintain accuracy or compatibility of data types between data sources and targets.
If done right, even after couple of transformations, one can say to an analytical metadata accepting function/algorithm: "get averages of this dataset" and it will understand it as "get averages of amounts in this dataset".
Basic metadata that should be considered when creating data processing or data analysing interfaces are:
- number of fields
- field names (as analyst I rather refer by name than index, as field position might differ among source chunks sometimes)
- field order (for tabular data it is implicit, for document based databases it should be specified)
- storage data types (at least generic, concrete if available or possible)
- analytical datatype
The minimal metadata structure for a dataset relevant to both: analysts who use data and engineers who prepare data would therefore be a list of tuples: (name, storage type, analytical type).
Conclusion
Typeless programming languages allow programmers to focus on structuring the data and remove the necessity of fiddling with physical storage implementation. Hiding concrete storage types from data analysts allows them to focus on properties of their data relevant to analysis. Less burden on mind definitely helps our thinking process.
Nevertheless, there are more kinds...
Links
Data Brewery documentation of metadata structures.